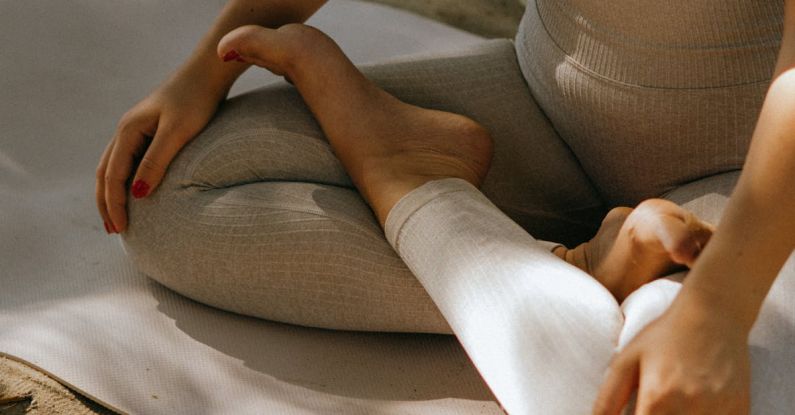
Data Cleaning Best Practices: Streamlining Your Data for Optimal Performance
In the world of data analysis and management, the process of data cleaning is crucial for ensuring the accuracy and reliability of the information being used. Data cleaning involves identifying and correcting errors or inconsistencies in the data to improve its quality and usefulness. By implementing best practices for data cleaning, organizations can streamline their data processes and make more informed decisions based on reliable data. This article will explore some of the key practices that can help organizations effectively clean their data.
Understanding the Importance of Data Cleaning
Before delving into the best practices for data cleaning, it is essential to understand why this process is so important. Data cleaning plays a critical role in ensuring the integrity of the data being used for analysis and decision-making. Without proper cleaning, data can be riddled with errors, duplicates, and inconsistencies, leading to inaccurate results and flawed conclusions.
By implementing best practices for data cleaning, organizations can improve the quality of their data, enhance the efficiency of their data analysis processes, and ultimately make more informed decisions based on reliable information.
Identifying and Removing Duplicate Data
One of the most common issues in data sets is the presence of duplicate entries. Duplicate data can skew analysis results and lead to inaccurate conclusions. To address this issue, organizations should implement processes to identify and remove duplicate data from their datasets.
Using data cleaning tools and techniques, organizations can easily identify duplicate entries based on specific criteria, such as matching values in key fields. Once duplicates are identified, they can be removed to ensure that the dataset is clean and free of redundant information.
Standardizing Data Formats and Values
Another important best practice for data cleaning is standardizing data formats and values across the dataset. Inconsistent data formats and values can lead to errors in analysis and hinder data integration efforts. By standardizing data formats and values, organizations can ensure that data is uniform and consistent, making it easier to analyze and use effectively.
To standardize data formats and values, organizations can create data validation rules and use data cleaning tools to automatically correct inconsistencies. By establishing clear standards for data formats and values, organizations can improve the quality and reliability of their data.
Cleaning Missing or Invalid Data
Missing or invalid data can significantly impact the accuracy of data analysis results. To address this issue, organizations should implement processes to clean missing or invalid data from their datasets. This can involve identifying missing values, outliers, or incorrect data entries and either removing them or replacing them with accurate information.
Data cleaning tools can help automate the process of identifying and cleaning missing or invalid data, making it easier for organizations to ensure the integrity of their datasets. By cleaning missing or invalid data, organizations can improve the quality and reliability of their data analysis results.
Ensuring Data Consistency and Accuracy
Maintaining data consistency and accuracy is essential for effective data cleaning. Organizations should establish clear data quality standards and implement processes to ensure that data is accurate, consistent, and reliable. This can involve regular data audits, data validation checks, and ongoing data cleaning efforts to identify and correct errors or inconsistencies.
By prioritizing data consistency and accuracy, organizations can improve the quality of their data and enhance the reliability of their analysis results. This can lead to more informed decision-making and better outcomes for the organization as a whole.
Embracing Automation and Technology
In today’s data-driven world, automation and technology play a crucial role in streamlining data cleaning processes. Organizations can leverage data cleaning tools and software to automate repetitive tasks, identify errors, and clean data more efficiently. By embracing automation and technology, organizations can save time and resources while improving the quality of their data.
From data deduplication tools to data validation software, there are numerous technologies available to help organizations streamline their data cleaning efforts. By leveraging these tools, organizations can enhance the accuracy and reliability of their data, making it easier to analyze and use effectively.
Conclusion: Elevating Data Quality Through Effective Data Cleaning Practices
Data cleaning is a critical process for ensuring the accuracy and reliability of data used for analysis and decision-making. By implementing best practices for data cleaning, organizations can streamline their data processes, improve data quality, and make more informed decisions based on reliable information.
From identifying and removing duplicate data to standardizing data formats and values, each best practice plays a key role in enhancing the quality of data. By prioritizing data consistency, accuracy, and automation, organizations can elevate their data quality and drive better outcomes for their business.
By embracing these best practices and making data cleaning a priority, organizations can harness the power of clean, reliable data to make more informed decisions and achieve their goals effectively.