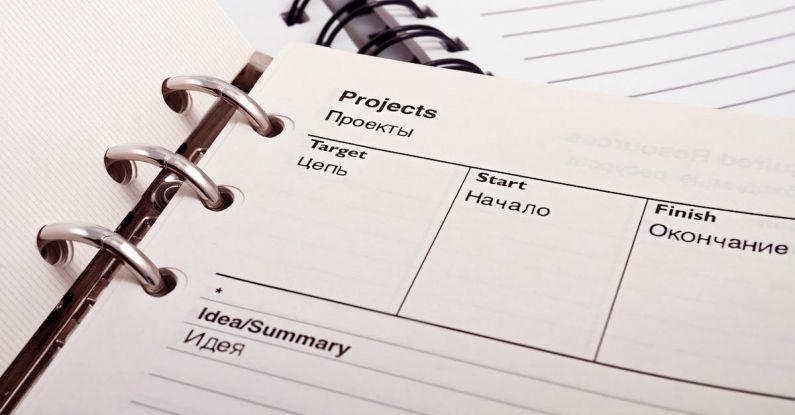
Data science projects have become increasingly popular in various industries, revolutionizing the way businesses operate and make decisions. However, with the vast amount of data available today, data science projects come with their own set of challenges that organizations must navigate to ensure successful outcomes. From data quality issues to resource constraints, these challenges can impact the effectiveness and efficiency of data science initiatives. In this article, we will explore some of the key challenges faced in data science projects and discuss strategies to overcome them.
Data Quality and Quantity
One of the primary challenges in data science projects is ensuring the quality and quantity of data. Poor data quality can lead to inaccurate results and flawed insights, ultimately impacting the decision-making process. Additionally, the sheer volume of data available can be overwhelming, making it difficult to identify relevant information and extract meaningful insights. To address these challenges, organizations must invest in data cleansing and preprocessing techniques to ensure the accuracy and reliability of their data. By implementing data quality checks and validation processes, organizations can improve the overall quality of their data and enhance the effectiveness of their data science projects.
Data Privacy and Security
Data privacy and security are major concerns in data science projects, especially with the increasing focus on data protection regulations such as GDPR and CCPA. Organizations must ensure that they are compliant with these regulations and take appropriate measures to safeguard sensitive data from unauthorized access and breaches. Implementing encryption techniques, access controls, and data anonymization methods can help mitigate the risks associated with data privacy and security. By prioritizing data protection measures, organizations can build trust with their customers and stakeholders and uphold their reputation in the market.
Resource Constraints
Resource constraints, including budget limitations and talent shortages, can pose significant challenges in data science projects. Building an effective data science team requires a diverse set of skills, including data analysis, machine learning, and programming expertise. However, finding and retaining top talent in the field of data science can be challenging, particularly for smaller organizations with limited resources. To overcome resource constraints, organizations can leverage external partnerships, collaborate with academic institutions, and invest in training and development programs to upskill existing employees. By expanding their talent pool and fostering a culture of continuous learning, organizations can address the resource constraints and enhance the capabilities of their data science teams.
Data Integration and Compatibility
Data integration and compatibility issues are common challenges in data science projects, particularly when dealing with disparate data sources and formats. Integrating data from multiple sources and ensuring compatibility across different systems can be complex and time-consuming, leading to delays in project timelines and inefficiencies in data processing. To address these challenges, organizations can implement data integration tools and technologies that facilitate seamless data exchange and interoperability. By standardizing data formats, establishing data governance frameworks, and adopting data integration best practices, organizations can streamline the data integration process and improve the overall efficiency of their data science projects.
Conclusion
In conclusion, data science projects present a myriad of challenges that organizations must navigate to achieve successful outcomes. From data quality issues to resource constraints, data privacy concerns, and data integration challenges, organizations must be proactive in addressing these obstacles to unlock the full potential of their data science initiatives. By investing in data quality measures, prioritizing data privacy and security, overcoming resource constraints, and optimizing data integration processes, organizations can enhance the effectiveness and efficiency of their data science projects and drive value for their business.